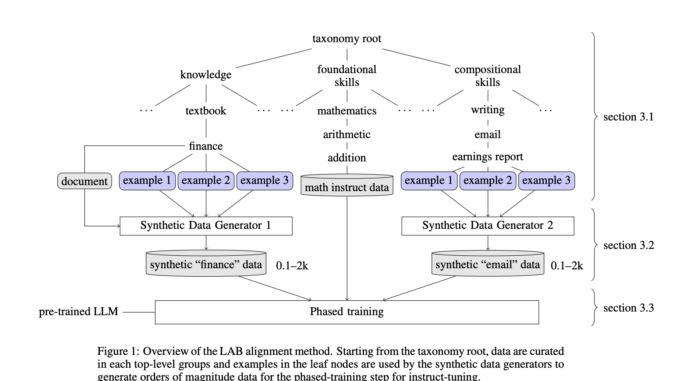
[ad_1]
IBM researchers have introduced LAB (Large-scale Alignment for chatbots) to address the scalability challenges encountered during the instruction-tuning phase of training large language models (LLMs). While LLMs have revolutionized natural language processing (NLP) applications, the instruction-tuning phase and fine-tuning of the models for specific tasks require high resource requirements and are highly dependable on human annotations and proprietary models like GPT-4. This requirement presents challenges in cost, scalability, and access to high-quality training data.
Currently, instruction tuning involves training LLMs on specific tasks using human-annotated data or synthetic data generated by pre-trained models like GPT-4. These methods are expensive, not scalable, and may not be able to retain knowledge and adapt to new tasks. To address these challenges, the paper introduces LAB (Large-scale Alignment for chatbots), a novel methodology for instruction tuning. LAB leverages a taxonomy-guided synthetic data generation process and a multi-phase tuning framework to reduce reliance on expensive human annotations and proprietary models. This approach aims to enhance LLM capabilities and instruction-following behaviors without the drawbacks of catastrophic forgetting, offering a cost-effective and scalable solution for training LLMs.
LAB consists of two main components: a taxonomy-driven synthetic data generation method and a multi-phase training framework. The taxonomy organizes tasks into knowledge, foundational skills, and compositional skills branches, allowing for targeted data curation and generation. Synthetic data generation is guided by the taxonomy to ensure diversity and quality in the generated data. The multi-phase training framework comprises knowledge tuning and skills tuning phases, with a replay buffer to prevent catastrophic forgetting. Empirical results demonstrate that LAB-trained models achieve competitive performance across several benchmarks compared to models trained with traditional human-annotated or GPT-4 generated synthetic data. LAB is evaluated by six different metrics, including MT-Bench, MMLU, ARC, HellaSwag, Winograde, and GSM8k, and the results demonstrate that LAB-trained models perform competitively across a wide range of natural language processing tasks, outperforming previous models’ fine-tuned by Gpt-4 or human-annotated data. LABRADORITE-13B and MERLINITE-7B, aligned using LAB, outperform existing models regarding chatbot capability while maintaining knowledge and reasoning capabilities.
In conclusion, the paper introduces LAB as a novel methodology to address the scalability challenges in instruction tuning for LLMs. LAB offers a cost-effective and scalable solution for enhancing LLM capabilities without catastrophic forgetting by leveraging taxonomy-guided synthetic data generation and a multi-phase training framework. The proposed method achieves state-of-the-art performance in chatbot capability while maintaining knowledge and reasoning capabilities. LAB represents a significant step forward in the efficient training of LLMs for a wide range of applications.
Check out the Paper and Blog. All credit for this research goes to the researchers of this project. Also, don’t forget to follow us on Twitter. Join our Telegram Channel, Discord Channel, and LinkedIn Group.
If you like our work, you will love our newsletter..
Don’t Forget to join our 38k+ ML SubReddit
Pragati Jhunjhunwala is a consulting intern at MarktechPost. She is currently pursuing her B.Tech from the Indian Institute of Technology(IIT), Kharagpur. She is a tech enthusiast and has a keen interest in the scope of software and data science applications. She is always reading about the developments in different field of AI and ML.
[ad_2]
Source link
Be the first to comment