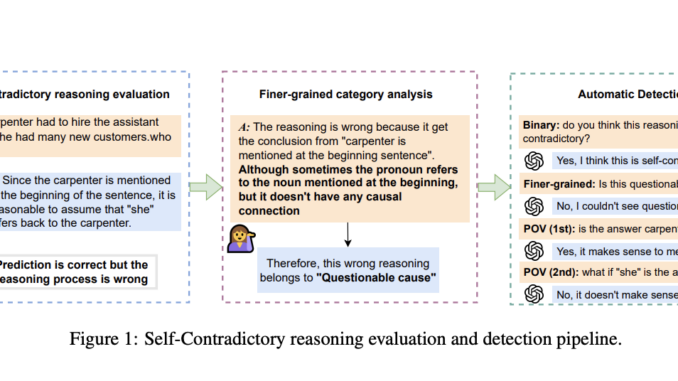
[ad_1]
Large language models, or LLMs, have transformed how machines understand and generate text, making interactions increasingly human-like. These models are at the forefront of technological advancements, tackling complex tasks from answering questions to summarizing vast amounts of text. Despite their prowess, a pressing question looms over their reasoning abilities: How reliable and consistent are they in their logic and conclusions?
A particular area of concern is self-contradictory reasoning, a scenario where the model’s logic does not align with its conclusions. This discrepancy raises doubts about the soundness of the models’ reasoning capabilities, even when they churn out correct answers. Traditional evaluation metrics focused heavily on outcomes like accuracy fall short of scrutinizing the reasoning process. This oversight means that a model might be rewarded for the right answers, which were arrived at through flawed logic, thereby masking the underlying issues in reasoning consistency.
Researchers from the University of Southern California have introduced a novel approach to scrutinize and detect instances of self-contradictory reasoning in LLMs to address this gap. This method goes beyond surface-level performance indicators, delving into the models’ reasoning processes to identify inconsistencies. It categorizes these inconsistencies, offering a granular view of where and how models’ logic falters. This approach is a significant leap forward, promising a more holistic evaluation of LLMs by spotlighting the alignment, or lack thereof, between their reasoning and predictions.
The methodology assesses reasoning across various datasets, pinpointing inconsistencies that previous metrics might overlook. This evaluation is crucial in understanding how much models can be trusted to make logical, consistent conclusions. Particularly, the study harnesses the power of GPT-4, among other models, to probe the depths of reasoning quality. It carefully examines different reasoning errors, classifying them into distinct categories. This classification illuminates the specific areas where models struggle and sets the stage for targeted improvements in model training and evaluation practices.
Despite achieving high accuracy on numerous tasks, LLMs, including GPT-4, demonstrate a propensity for self-contradictory reasoning. This alarming observation indicates that models often resort to incorrect or incomplete logic pathways to arrive at correct answers. Such a paradox underscores a critical flaw in relying solely on outcome-based evaluation metrics like accuracy, which can obscure the underlying reasoning quality of LLMs. This discovery calls for a paradigm shift in how we assess and understand the capabilities of these advanced models.
The study’s performance evaluation and detection of self-contradictory reasoning highlight the urgent need for more nuanced and comprehensive evaluation frameworks. These frameworks must prioritize the integrity of reasoning processes, ensuring that models are accurate, logically sound, and reliable. The research points to a significant gap in current evaluation methods, advocating for a holistic approach that considers the correctness of answers and the logical coherence of the reasoning leading to those answers.
In conclusion, this research casts a spotlight on the critical issue of self-contradictory reasoning in LLMs, urging a reevaluation of how we gauge these models’ capabilities. Proposing a detailed framework for assessing reasoning quality paves the way for more reliable and consistent AI systems. This endeavor is about critiquing current models and laying the groundwork for future advancements. It is a call to action for researchers and developers to prioritize logical consistency and reliability in the next generation of LLMs, ensuring they are powerful and trustworthy.
Check out the Paper. All credit for this research goes to the researchers of this project. Also, don’t forget to follow us on Twitter and Google News. Join our 38k+ ML SubReddit, 41k+ Facebook Community, Discord Channel, and LinkedIn Group.
If you like our work, you will love our newsletter..
Don’t Forget to join our Telegram ChannelYou may also like our FREE AI Courses….
Hello, My name is Adnan Hassan. I am a consulting intern at Marktechpost and soon to be a management trainee at American Express. I am currently pursuing a dual degree at the Indian Institute of Technology, Kharagpur. I am passionate about technology and want to create new products that make a difference.
[ad_2]
Source link
Be the first to comment